MSTYLEDISTANCE: Multilingual Style Embeddings and their Evaluation
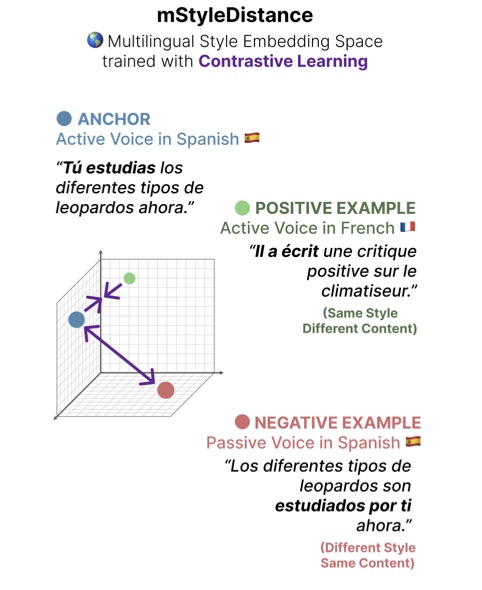
We introduce Multilingual STYLE DISTANCE, expanding our prior study STYLE DISTANCE to a multilingual scenario.
Expanding content-independent Style Representation to a multilingual setting
Style embeddings are useful for stylistic analysis and style transfer; however, only English style embeddings have been made available. We introduce Multilingual STYLEDISTANCE (MSTYLEDISTANCE), a multilingual style embedding model trained using synthetic data and contrastive learning. We train the model on data from nine languages and create a multilingual STEL-or-Content benchmark (Wegmann et al., 2022) that serves to assess the embeddings’ quality. We also employ our embeddings in an authorship verification task involving different languages. Our results show that MSTYLEDISTANCE embeddings outperform existing models on these multilingual style benchmarks and generalize well to unseen features and languages.
Key Contributions
- Synthetic Multilingual Dataset: We created a dataset of paraphrases addressing various style features in nine languages, and use it to create our multilingual style embeddings
- MSTYLEDISTANCE: use Synthetic Multilingual Dataset to create our multilingual style embeddings, which outperform other representations on STEL-or-Content (SoC) evaluation, and demonstrate their usefulness in a downstream setting addressing a multilingual authorship verification task.
Access the Model
- For those interested in exploring or utilizing our model, it is available at mstyledistance
- Synthetic Dataset is available at msynthstel
- Paper is available at ArXiv Link