StyleDistance: Stronger Content-Independent Style Embeddings with Synthetic Parallel Examples
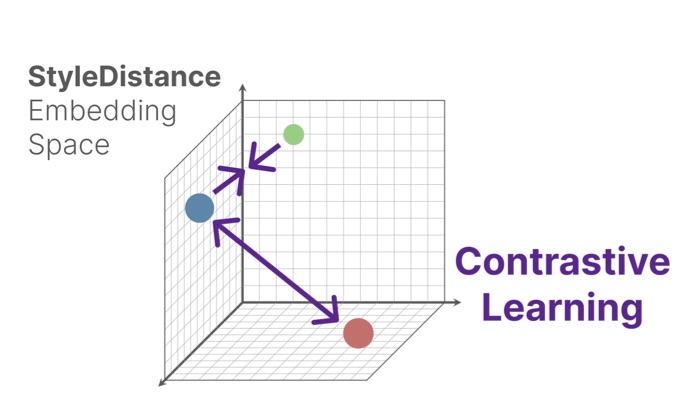
We introduce STYLE DISTANCE, a novel method for training style representations that effectively separate writing style from content by leveraging a synthetic dataset of paraphrases with controlled style variations. Our approach achieves superior content-independent embeddings, validated through human and automated evaluations, and outperforms existing methods on real-world benchmarks.
Addressing Content Leakage in Style Representations
Traditional methods for developing style representations often encounter the issue of content leakage, where embeddings inadvertently capture content-specific information, compromising their effectiveness. To tackle this challenge, we introduce StyleDistance, a novel approach that leverages large language models to generate synthetic datasets comprising near-exact paraphrases with controlled stylistic variations. This methodology enables precise contrastive learning across 40 distinct style features, significantly enhancing the content-independence of style embeddings.
Key Contributions
- Synthetic Dataset Generation: We created a dataset of near-exact paraphrases, each exhibiting controlled stylistic variations, facilitating precise contrastive learning.
- Enhanced Content-Independence: By focusing on style features while controlling for content, StyleDistance achieves superior separation between style and content in embeddings.
- Robust Evaluation: Our approach was rigorously evaluated through both human assessments and automated benchmarks, demonstrating its effectiveness in real-world applications.
Access the Model
- For those interested in exploring or utilizing our model, it is available at styledistance
- Synthetic Dataset is available at synthstel
- Paper is available at ArXiv Link